
Machine Learning-Connecting Present Through Future

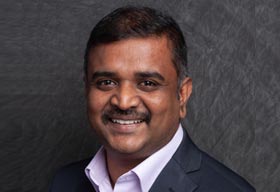
Machine learning has become a buzz word today with most software products or services claiming that they are powered by machine learning. In the hype cycle, machine learning is reaching the peak of inflated expectations. As a result of this, there is a widespread belief that machine learning is a silver bullet to all problems. While some of it may not be realistic, at least with current technology, it does offer very interesting possibilities that we could not have imagined a decade ago.
Demystifying Machine Learning
Machine learning is not a new technology breakthrough, it has been here for many decades. It is getting more attention now as it has become commercially viable to implement applications with machine learning abilities, thanks to advancements in computing technology. If you are new to machine learning, you should understand the fundamental paradigm shift in how traditional programs were written and how a machine learning-based program is written.
Traditionally programs were given a set of inputs. Rules are pre-coded into them which when applied over the inputs gives the result. A machine learning program takes in a set of inputs and the corresponding outputs, it then figures out the rules of the program that when applied to the input gives the corresponding output.

In the example illustrated in the figure, the machine learning program makes an educated guess of the rules when applied to the inputs to deliver a certain output. In reality, the rule is not as simple as that of a sum, it could be a linear regression model. One advantage of letting a program predict the rules is that the program can adapt itself to the changing behaviors of inputs and outputs to appropriately determine the new rules. How close the outputs are when applied to the inputs determines the accuracy of the machine learning program. The program when determining the rules checks the accuracy of the outcome which is known as feedback. Based on the feedback it can refine the rules until the desired accuracy is achieved. This iterative process of determining the rules is called machine learning.

Accuracy is usually denoted as a percentage. Once the program achieves the desired accuracy it stops learning. It now has a model that can predict the output for a new set of inputs. The words accuracy and predict are used, as there is always room for error. This is what makes a machine learning program
Prediction of the Future
The design of the machine learning program to create rules for prediction has numerous applications. Some of them are listed below and definitely not limited to these.
1. Based on the shopping patterns of a customer on a retail website, newer product recommendations which have a high likelihood of being purchased are shown to the customer. The rules for the "likelihood of being purchased" are determined by a machine learning program. These rules adapt to customers changing needs. An exam-example is that the rules that apply when the customer is single are different from when the customer is in a relationship which are different when the customer is a parent. Machine learning learns the new rules based on changes in behaviors.
The only thing you must remember before spreading your wings or going for a broader spectrum with multiple specializations in content is, never should your plans affect the value of your current product
2. Techniques in machine learning, like deep learning, enable the ability to relate unrelated factors that affect the outcome. For example, a deep learning algorithm can recommend a shoe for a customer based on a rich feature set which includes, the type of material, color, the narrowness of the tip, the height of the heels, the grip of the sole, patterns on the front and many more factors.
3. Course recommendation systems can help students determine the courses in which they have a better probability of being successful. Factors considered in this algorithm are time if day, professor, work schedule, and more.
4. Recruiters can use machine learning programs that sort resumes based on the likelihood of a candidate to succeed based on the job requirements for the role and the candidates past experience.
5. Automobile companies can use machine learning programs that can predict the wearing of spare parts based on the past data of spare part replacement. Analyzing under what circumstances the spare parts were replaced in the past would help in identifying and replacing the spare parts before they are damaged thereby reducing breakdowns.
In all of the above examples, the outcome of the programs is a prediction. Machine learning programs can be seen as a predictive analytics system that can continuously adapt itself to the changing nature and behavior of the inputs and the outputs. Traditional analytics systems derive information from the data available, while a machine learning-powered predictive analytics system helps in predicting an outcome based on the data available. The traditional analytics systems provide information for deciding the course of action, while a machine learning-powered predictive analytics system recommends the course of action. Thus, machine learning programs connect the present to the future.
Word of Caution
Before going all out for machine learning there is certain caution that needs to be exercised.
1. The prediction of the outcome is only as good as the data that is available. Even a great algorithm when operating on poor data can produce poor predictions.
2. 100 per cent accurate machine learning programs suffer from a phenomenon of “over-fitting” wherein it works only with the data used for training.
3. There is a risk of bias in machine learning programs. It has the vulnerability of taking in biased data and produce a biased outcome. Hackers can exploit the nature of the prediction systems to change the outcomes. Hence the data and rules need to be kept transparent to gain more trust in the machine learning program.
Before concluding it is necessary to touch upon the dreaded outcome of machine learning. Can machines replace humans? The answer is “No, not yet”. Technology is far from what has been portrayed in movies where machines take over human roles. However, machine learning programs certainly increase the humans’ dependency on machines more than ever and when used wisely can help in a constructive manner.