
Next-Gen Cyber Security With AI

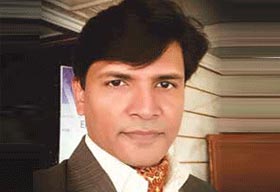
The landscape of IT security has been continuously evolving. Industry has shifted gears from Preventive security measures to Predictive measures and now moving towards Perspective models. The Cyber security solutions fuelled with Artificial Intelligence is taking the centre stage. Cybersecurity solutions that use Artificial Intelligence and Machine Learning typically use historical data from prior cyber-events to dynamically detect and respond to newer but somewhat similar attacks. It means using machine learning to find anomalies. i.e. to identify malicious behaviour or malicious entities against normal patterns. However, one of the key challenges is to define what is normal.
AI considerations need to be applied for both the aspects of security, threat detection and threat response. The degree of maturity of any security solution depends on time taken to detect the threat and time taken to respond back. Broadly, I would look at three levels of maturity, for threat detection, first level being anticipating threat even before it strikes, second level looks at hunting forevasive threats and third level is threat monitoring to detect known threats. Similarly, for response, the levels of maturity can be defined as
deliberated response, immediate response and a self-healing system.
Based on ESG research 29 percent of security professionals, intend to use AI-based cybersecurity solutions to accelerate incident detection. If we take a closer look at role of AI in threat detection, it becomes important to identify data patterns and baseline them. This means to collect validated and curated threat intelligence data like malicious IPs, URLs, files, hashes, processes, and signatures, in a machine-readable format and derive patterns and anti-patterns. An advanced deep-learning solution would have the capability to dynamically update these baseline patterns as well. Keys areas that needs to be considered for threat modeling and analytics are application-level, Network, End-points, and User behavioural patterns.
The type of Machine Learning, one adopts is based on the use-case one is trying to address. Supervised machine learning is applicable, where one has large amount of labelled samples and it is a straight fit for use-cases like spam detection, malware classification, classification of files. However practically it is not easy to assemble a good training data set and without which training the algorithm for accuracy becomes difficult.
Unsupervised machine learning can help clubbing the set of data records and detect anomalies. Dimensionality reduction, clustering, association rules are various methods, which helps in anomaly detection, data exploration, entity classification. These methods would help diagnose threats related to network traffic, abnormal entities like users, devices, servers. Some fundamental problems that need to be addressed while devising these solutions are distance functions and expansion of the clusters and hence dealing with false positives.
Based on ESG research 29 percent of security professionals, intend to use AI-based cybersecurity solutions to accelerate incident detection. If we take a closer look at role of AI in threat detection, it becomes important to identify data patterns and baseline them. This means to collect validated and curated threat intelligence data like malicious IPs, URLs, files, hashes, processes, and signatures, in a machine-readable format and derive patterns and anti-patterns. An advanced deep-learning solution would have the capability to dynamically update these baseline patterns as well. Keys areas that needs to be considered for threat modeling and analytics are application-level, Network, End-points, and User behavioural patterns.
Around 27 percent of security professional, as per the ESG research, want to use AI-based cybersecurity technology to accelerate incident response. Use of AI in responding to threats, means a lot of automation in forensics and investigation, Impact-analysis modelling to promptly handle incidents, minimizing the impact and finally automation of response based on playbooks and autonomic computing principles. Advanced use of autonomic computing enables development of full-scale self-healing system, with minimal human intervention.AI considerations need to be applied for both the aspects of security, threat detection and threat response
The type of Machine Learning, one adopts is based on the use-case one is trying to address. Supervised machine learning is applicable, where one has large amount of labelled samples and it is a straight fit for use-cases like spam detection, malware classification, classification of files. However practically it is not easy to assemble a good training data set and without which training the algorithm for accuracy becomes difficult.
Unsupervised machine learning can help clubbing the set of data records and detect anomalies. Dimensionality reduction, clustering, association rules are various methods, which helps in anomaly detection, data exploration, entity classification. These methods would help diagnose threats related to network traffic, abnormal entities like users, devices, servers. Some fundamental problems that need to be addressed while devising these solutions are distance functions and expansion of the clusters and hence dealing with false positives.